2401 02777 From LLM to Conversational Agent: A Memory Enhanced Architecture with Fine-Tuning of Large Language Models

Overall, conversational AI apps have been able to replicate human conversational experiences well, leading to higher rates of customer satisfaction. Natural language processing is the current method of analyzing language with the help of machine learning used in conversational AI. Before machine learning, the evolution of language processing methodologies went from linguistics to computational linguistics to statistical natural language processing.
Data security is an uncompromising aspect and we should adhere to best security practices for developing and deploying conversational AI across the web and mobile applications. Having proper authentication, avoiding any data stored locally, and encryption of data in transit and at rest are some of the basic practices to be incorporated. Also understanding the need for any third-party integrations to support the conversation should be detailed.
Architecture of CoRover Platform is Modular, Secure, Reliable, Robust, Scalable and Extendable. Our innovation in technology is the most unique property, which makes us a differential provider in the market. Assisted Learning
Analytics outputs can be used to improve a Virtual Agent’s performance. Backend Integrations
CAIP is designed with support for enterprise level backend integration in mind. Leverage existing investment
Unify previously siloed initiatives and build on various technologies without needing to rebuild from scratch. Logging and analytics tools better enable operations and maintenance, creating a living system.
Conversational AI and equity through assessing GPT-3’s communication with diverse social groups on contentious … – Nature.com
Conversational AI and equity through assessing GPT-3’s communication with diverse social groups on contentious ….
Posted: Thu, 18 Jan 2024 08:00:00 GMT [source]
As customer satisfaction grows, companies will see its impact reflected in increased customer loyalty and additional revenue from referrals. Human conversations can also result in inconsistent responses to potential customers. Since most interactions with support are information-seeking and repetitive, businesses can program conversational AI to handle various use cases, ensuring comprehensiveness and consistency. This creates continuity within the customer experience, and it allows valuable human resources to be available for more complex queries. Staffing a customer service department can be quite costly, especially as you seek to answer questions outside regular office hours.
1 How does natural language processing (NLP) work?
If the template requires some placeholder values to be filled up, those values are also passed by the dialogue manager to the generator. Then the appropriate message is displayed to the user and the bot goes into a wait mode listening for the user input. Picture a scenario where the model is given an incomplete sentence, and its task is to fill in the missing words. Thanks to the knowledge amassed during pre-training, LLM Chatbot Architecture can predict the most likely words that would fit seamlessly into the given context. In this blog, we will explore how LLM Chatbot Architecture contribute to Conversational AI and provide easy-to-understand code examples to demonstrate their potential.
In this codelab, we’ll focus on building the shopping cart experience and deploying the application to Google App Engine. Furthermore, chatbots can integrate with other applications and systems to perform actions such as booking appointments, making reservations, or even controlling smart home devices. The possibilities are endless when it comes to customizing chatbot integrations to meet specific business needs.
Robust analytics dashboard and extensive reports to track conversation performance. Language generation models like GPT-3 and BARD are computationally intensive, requiring significant GPU resources for inference. Strategies such as model quantization, distillation, and efficient batching can help reduce computational costs and enable scalable deployment.
- Natural language processing strives to build machines that understand text or voice data, and respond with text or speech of their own, in much the same way humans do.
- Each block input is tightly connected to the last subblock of all following blocks, using a dense residual connection (to learn more about residual nets, check this article).
- In addition to Apple’s own homegrown AI tech, the company’s phones, computers and iPads will also have ChatGPT built in “later this year,” a huge validation of the importance of the highflying start-up’s tech.
In summary, well-designed backend integrations make the AI assistant more knowledgeable and capable. It may be the case that UI already exists and the rules of the game have just been handed over to you. For instance, building an action for Google Home means the assistant you build simply needs to adhere to the standards of Action design.
CNN uses convolution layers to apply convolution operations to the input data to extract features. After convolution, pooling layers are used to reduce the spatial dimensions of the data. It involves down sampling the feature maps created by the convolutional layers, by taking max-pooling and average-pooling. After convolutional and pooling layers, activation functions are applied to introduce non-linearity into the network [16].
How different is it from say telephony that also supports natural human-human speech? Understanding the UI design and its limitations help design the other components of the conversational experience. They can consider the entire conversation history to provide relevant and coherent responses. In the past, interacting with chatbots often felt like talking to a preprogrammed machine. These rule-based bots relied on strict commands and predefined responses, unable to adapt to the subtle nuances of human language. Users often hit dead ends, frustrated by the bot’s inability to comprehend their queries, and ultimately dissatisfied with the experience.
Accenture’s Customer Engagement Conversational AI Platform (CAIP) relieves pressure on the contact center with self-service automation—powered by generative AI (GenAI)—to optimize the customer experience. While conversational AI offers several advantages, human intervention may still be necessary for complex or sensitive issues. It may not be suitable for all situations and may require careful design and monitoring to ensure a positive user experience.
“We can save a designer time by searching through all those sources to answer questions as specific as ‘what is the range of concrete costs that we’ve used on previous projects above $2 million in Illinois? WSP uses computer vision to automate dull data entry work such as vendor invoices and concrete mix certifications, leading to an immense increase in efficiency and quality control. In this course, learn to use additional features of Dialogflow ES for your virtual agent, create a Firestore instance to store customer data, and implement cloud functions that access the data. With the ability to read and write customer data, learner’s virtual agents are conversationally dynamic and able to defer contact center volume from human agents.
The ASRViewController, handling the API Callbacks
Artificial Intelligence (AI) powers several business functions across industries today, its efficacy having been proven by many intelligent applications. From healthcare to hospitality, retail to real estate, conversational ai architecture insurance to aviation, chatbots have become a ubiquitous and useful feature. User experience design is a established field of study that can provide us with great insights to develop a great experience.

This includes designing solutions to log conversations, extracting insights, visualising the results, monitoring models, resampling for retraining, etc. Designing an analytics solution becomes essential to create a feedback loop to make your AI powered assistant, a learning system. Many out of the box solutions are available — BotAnalytics, Dashbot.io, Chatbase, etc. The real breakthrough came with the emergence of Transformer-based models, notably the revolutionary GPT (Generative Pre-trained Transformer) series. GPT-3, the third iteration, represented a game-changer in conversational AI.
It’s based on principles of collaboration, unobstructed discovery, and, most importantly, scientific progression. As PhD students, we found it difficult to access the research we needed, so we decided to create a new Open Access publisher that levels the playing field for scientists across the world. By making research easy to access, and puts the academic needs of the researchers before the business interests of publishers. When developing conversational AI you also need to ensure easier integration with your existing applications.
When people think of conversational artificial intelligence, online chatbots and voice assistants frequently come to mind for their customer support services and omni-channel deployment. Most conversational AI apps have extensive analytics built into the backend program, helping ensure human-like conversational experiences. The technology choice is also critical and all options should be weighed against before making a choice. Each solution has a way of defining and handling the conversation flow, which should be considered to decide on the same as applicable to the domain in question.
Here we will use GPT-3.5-turbo, an example of llm for chatbots, to build a chatbot that acts as an interviewer. The llm chatbot architecture plays a crucial role in ensuring the effectiveness and efficiency of the conversation. These models utilized statistical algorithms to analyze large text datasets and learn patterns from the data. With this approach, chatbots could handle a more extensive range of inputs and provide slightly more contextually relevant responses. However, they still struggled to capture the intricacies of human language, often resulting in unnatural and detached responses. With Neural Modules, they wanted to create general-purpose Pytorch classes from which every model architecture derives.
It took 19 steps of nanofabrication to get the diamond quantum microchiplets, and the steps were not straightforward,” he adds. The researchers demonstrated a 500-micron by 500-micron area transfer for an array with 1,024 diamond Chat GPT nanoantennas, but they could use larger diamond arrays and a larger CMOS chip to further scale up the system. In fact, they found that with more qubits, tuning the frequencies actually requires less voltage for this architecture.
CNNs are a class of Deep Neural Networks that can recognize and classify particular features. Convolution is a mathematical process that involves multiplying two functions to create a third function that expresses how the form of one function is altered by the other. The term “convolution” is used in CNN to refer to this mathematical activity [15].
Let’s dive in and see how LLMs can make our virtual interactions more engaging and intuitive. Machine learning is a branch of artificial intelligence (AI) that focuses on the use of data and algorithms to imitate the way that humans learn. Your FAQs form the basis of goals, or intents, expressed within the user’s input, such as accessing an account.
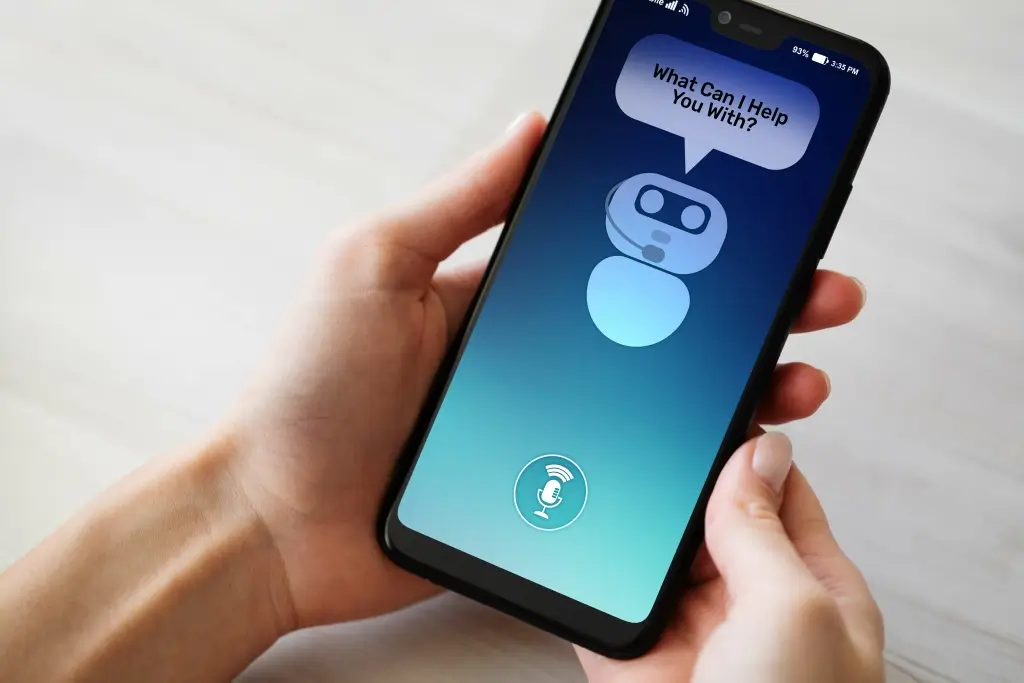
It ensures that conversational AI models process the language and understand user intent and context. For instance, the same sentence might have different meanings based on the context in which it’s used. You can use conversational AI solutions to streamline your customer service workflows.
Whether you’re looking for a ready-to-use product or decide to build a custom chatbot, remember that expert guidance can help. If you’d like to talk through your use case, you can book a free consultation here. Designing solutions that use of these models, orchestrate between them optimally and manage interaction with the user is the job of the AI designer/architect. In addition, these solutions need also be scalable, robust, resilient and secure. There are many principles that we can use to design and deliver a great UI — Gestalt principles to design visual elements, Shneiderman’s Golder rules for functional UI design, Hick’s law for better UX. The parameters such as ‘engine,’ ‘max_tokens,’ and ‘temperature’ control the behavior and length of the response, and the function returns the generated response as a text string.
No code platform
Conversational AI Virtual Agents can be designed, built, trained and integrated into backend services (using APIs) by business analysts without writing code. As an enterprise architect, it’s crucial to incorporate conversational AI into the organization’s tech stack to keep up with the changing technological landscape. Boards around the world are requiring CEOs to integrate conversational AI into every facet of their business, and this document provides a guide to using conversational AI in the enterprise. We’ll be using the Django REST Framework to build a simple API for serving our models. The idea is to configure all the required files, including the models, routing pipes, and views, so that we can easily test the inference through forward POST and GET requests. In addition, if we want to combine multiple models to build a more sophisticated pipeline, organizing our work is key to separate the concerns of each part, and make our code easy to maintain.
Even after all this, the chatbot may not have an answer to every user query. A document search module makes it possible for the bot to search through documents or webpages and come up with an appropriate answer. The architecture of a chatbot can vary depending on the specific requirements and technologies used. As chatbot technology continues to evolve, we can expect more advanced features and capabilities to be integrated, enabling chatbots to provide even more personalized and human-like interactions. AI tech is the central component in the design of a Conversational AI solution.
The UI/UX should be clearly defined for all possible flows and interactions. Like for any other product, it is important to have a view of the end product in the form of wireframes and mockups to showcase different possible scenarios, if applicable. For e.g. if your chatbot provides media responses in the form of images, document links, video links, etc., or redirects you to a different knowledge repository. Additionally, you can integrate past customer interaction data with conversational AI to create a personalized experience for your customers.
When the outcome is binary, such as the presence or absence of a disease (such as non-Hodgkin’s lymphoma), the model is referred to as a binary logistic model. This chapter looks at categorical, continuous, and multiple binary logistic regression models, as well as interaction, quality of fit, categorical predictor variables, and multiple predictor variables [8]. Open Access is an initiative that aims to make scientific research freely available to all.
Here the ‘if-this-then-that’ kind of rules work for addressing user queries. If certain required entities are missing in the intent, the bot will try to get those by putting back the appropriate questions to the user. Apparently most organizations that use chat and / or voice bots still make little use of conversational analytics. A missed opportunity, given the intelligent use of conversational analytics can help to organize relevant data and improve the customer experience.
Enterprises must closely track certain KPIs, such as response time, resolution rates, time to resolution, and feedback. RAG is a boon here, enabling organizations to refine the bot’s conversational quotient, knowledge, and decision-making abilities. A quick hack requires establishing a practice of feedback loops, enabling customers to report issues, suggest improvements, and deliver valuable insights. In early retrieval systems, TF-Id [42], bags of words, etc. were used as score functions in feature extraction. In recent years, deep learning has dominated a wide range of application fields. Deep learning classifiers enhance accuracy and performance by automatically learning and extracting information.
- Although these presumptions are generally not true in real-world situations, they make categorization problems more manageable from a computational standpoint.
- Through a combination of presentations, demos, and hands-on labs, participants learn how to create virtual agents.
- The app will be available starting on Monday, free of charge, for both smartphones and desktop computers.
- In recent years, deep learning has dominated a wide range of application fields.
Olujimi [54] has shown NLP-based enhancement and AI in business ecosystems in their research. This indicates NLP is one of the fast-growing research domains in AI, with a variety of applications. Conversational artificial intelligence (AI) refers to technologies, such as chatbots or virtual agents, that users can talk to. They use large volumes of data, machine learning and natural language processing to help imitate human interactions, recognizing speech and text inputs and translating their meanings across various languages. LLMs have significantly enhanced conversational AI systems, allowing chatbots and virtual assistants to engage in more natural, context-aware, and meaningful conversations with users.
Question-answer relevance is a measure of how relevant an answer is to the user’s query. The product of question-question similarity and question-answer relevance is the final score that the bot considers to make a decision. A BERT-based FAQ retrieval system is a powerful tool to query an FAQ page and come up with a relevant response. The module can help the bot answer questions even when they are worded differently from the expected FAQ.
If you are building an enterprise Chatbot you should be able to get the status of an open ticket from your ticketing solution or give your latest salary slip from your HRMS. In this codelab, you’ll learn how to integrate a simple Dialogflow Essentials (ES) text and voice bot into a Flutter app. To create a chatbot for mobile devices, you’ll have to create a custom integration. If the bot still fails to find the appropriate response, the final layer searches for the response in a large set of documents or webpages. It can find and return a section that contains the answer to the user query. We use a numerical statistic method called term frequency-inverse document frequency (TF-IDF) for information retrieval from a large corpus of data.
The library is robust, and gives a holistic tour of different deep learning models needed for conversational AI. Speech recognition, speech synthesis, text-to-speech to natural language processing, and many more. These use machine learning to map user utterances to intent and use rule based approach for dialogue management (e.g. DialogFlow, Watson, Luis, Lex, Rasa, etc). In addition to these, the understanding power of the assistant can be enhanced by using other NLP methods and machine learning models.
RNNs remember the previous computations and use this understanding of previous information in current processing. They can be used to handle a variety of tasks, including customer service, information retrieval, and language translation. The hidden layer contains a temporal feedback loop as shown in Figure 2 [29]. Particularly in conversation AI, sound waves are recognized into phonetic segments and subsequently joined together into words via the RNN application.

It should meet your customers, where they are, 24/7 and be proactive, ubiquitous, and scalable. Chatbots may seem like magic, but they rely on carefully crafted algorithms and technologies to deliver intelligent conversations. Now refer to the above figure, and the box that represents the NLU component (Natural Language Understanding) helps in extracting the intent and entities from the user request. The true prowess of Large Language Models reveals itself when put to the test across diverse language-related tasks. From seemingly simple tasks like text completion to highly complex challenges such as machine translation, GPT-3 and its peers have proven their mettle. Finally, conversational AI can also optimize the workflow in a company, leading to a reduction in the workforce for a particular job function.
Whether you want to chat with a Pokemon, George Washington, or Elon Musk, Character AI provides an interesting perspective that other chatbots can’t. You can engage in interesting conversations with AI-generated characters to expand your knowledge, provide inspiration, or be entertained. Unlike other AI chatbots, such as ChatGPT, Character AI’s output is more human-like and allows you to chat with more than one bot at a time, offering different perspectives. Developed by former Google AI developers Noam Shazeer and Daniel De Freitas, Character AI was released in beta form in September 2022.
You can foun additiona information about ai customer service and artificial intelligence and NLP. A pre-trained BERT model can be fine-tuned to create sophisticated models for a wide range of tasks such as answering questions and language inference, without substantial task-specific architecture modifications. It involves a sophisticated interplay of technologies such as Natural Language Processing, Machine Learning, and Sentiment Analysis. These technologies work together to create chatbots that can understand, learn, and empathize with users, delivering intelligent and engaging conversations. While chatbot architectures have core components, the integration aspect can be customized to meet specific business requirements. Chatbots can seamlessly integrate with customer relationship management (CRM) systems, e-commerce platforms, and other applications to provide personalized experiences and streamline workflows.
Revolutionizing AI Learning & Development
Writers that have great ideas but not the best writing style or grammar are making increasing use of AI to get real-time edits for grammar, style, and tone suggestions, making their written communication clearer and more polished. As previously mentioned, most of the output is likely false, so checking what it gives you is important. After playing with the Translator bot, we can say that it is mostly accurate and had no trouble translating a simple sentence into Urdu, the primary language spoken in Pakistan. Character AI isn’t just about conversing with celebrities or fictional entities.
With the GPU implementation of the ternary dense layers, they were able to accelerate training by 25.6% and reduce memory consumption by up to 61.0% over an unoptimized baseline implementation. Matrix multiplications (MatMul) are the most computationally expensive operations in large language models (LLM) using the Transformer architecture. As LLMs https://chat.openai.com/ scale to larger sizes, the cost of MatMul grows significantly, increasing memory usage and latency during training and inference. A 2016 study by the McKinsey Global Institute published in Harvard Business Review ranked the construction industry second to last in digital advancement, ahead of only “agriculture/hunting” of 22 industries studied.
Poliark introduces new generative AI-based design platform – Geo Week News
Poliark introduces new generative AI-based design platform.
Posted: Tue, 30 Jan 2024 08:00:00 GMT [source]
I mean, nothing to announce right now, but that’s our direction,” Federighi said. The company is already battling a Justice Department antitrust lawsuit that alleges it wields an illegal smartphone monopoly. Antitrust enforcers have been wary of the ways that tech companies use their deep war chests to strike deals that threaten innovation. Our community is about connecting people through open and thoughtful conversations. We want our readers to share their views and exchange ideas and facts in a safe space.
As part of the complete customer engagement stack, analytics is a very essential component that should be considered as part of the Conversational AI solution design. Having a complete list of data including the bot technical metrics, the model performance, product analytics metrics, and user feedback. Also, consider the need to track the aggregated KPIs of the bot engagement and performance.
Since it captures long-term dependencies, it facilitates the sequence prediction tasks. It is applied in conversational AI such as in tasks to predict the next word in the input sequence. This was the stepping stone to imbibe the functionality of remembering the conversation from the previous inputs in a dialog system [31]. It also belongs to the family of generative learning algorithms [9, 10], which model the input distribution of a certain class or category.
Handles all the logic related to voice recording using AVAudioRecorder shared instances, and setting up the internal directory path to save the generated audio file. We’ll be building the application programmatically, without using a storyboard, which means no boxes or buttons to toggle — just pure code. The same goes with the tts_transcription post method, where we run inference on input text to generate an output audio file with a sampling rate of 22050, and we save it with the write(path) method locally in the file system. But before actually implementing the API view, we need to instantiate model handlers in the global scope of the project, so that heavy config files and checkpoints can be loaded into memory and prepared for usage. If we’re employing the model in a sensitive scenario, we must chain the textual raw output from the ASR model with a punctuator, to help clarify the context and enhance readability.